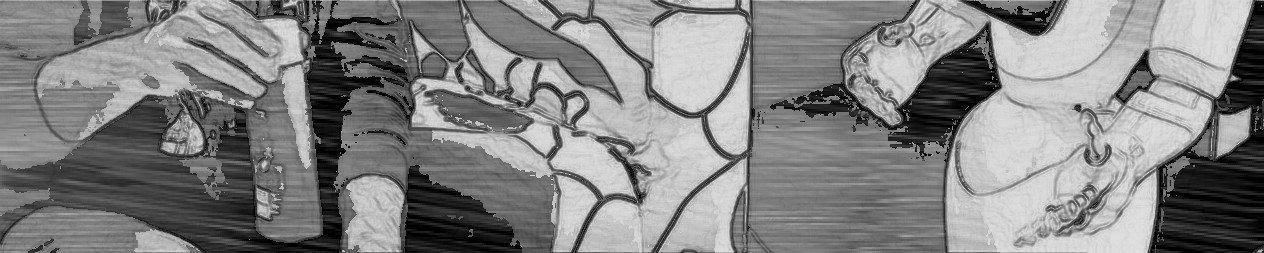
Workshop on Cognitive Architectures for HRI: Embodied Models of Situated Natural Language Interactions
(MM-Cog)
Program
14:00-14:05 Welcome
14:05-14:50 Keynote Chen Yu
14:50-15:03 When your face and tone of voice don't say it all: Inferring emotional state from word semantics and conversational topics (Andrew Valenti) [pdf]
15:03-15:16 Active language learning inspired from early childhood information seeking strategies (Christiana Tsiourti) [pdf]
15:16-15:30 The necessity of enactive simulation for linguistic comprehension (Ameer Sarwar) [pdf]
1/2h break
16:00-16:45 Keynote John Laird
16:45-16:58 Merging representation and management of physical and spoken action (Charles Threlkeld) [pdf]
16:58-17:11 Towards self-explaining social robots: verbal explanation strategies for a needs-based architecture (Sonja Stange) [pdf]
17:11-18:00 Discussion panel
Invited Talks
Language learning through Embodied Multimodal Interactions
Chen Yu
Professor of Psychological and Brain Sciences, Cognitive Science and Informatics
Indiana University
Abstract
Interacting embodied agents, be they groups of adult humans engaged in a coordinated task, autonomous robots acting in an environment, or a mother teaching a child, must seamlessly coordinate their actions to achieve a collaborative goal. Inter-agent coordination depends crucially on external behaviors by the participants where the behavior of one participant organizes the actions of the other in real time. In this talk, I will review a set of studies using a novel experimental paradigm in which we collect high-density multimodal behavioral data (including eye tracking, motion tracking, audio and video) in both parent-child and human-robot interactions. We compare and analyze the dynamic structure of free-flowing parent-child and human-robot interactions in the context of language learning, and discover the characteristics of the learning agents perceptual, attentional and motor systems in such interactions, and as well as perceptual and motor patterns that are informatively time-locked to words and their intended referents and predictive of word learning.
Chen Yu is Professor of Psychological and Brain Sciences, Cognitive Science and Informatics at the Computational Cognition and Learning Lab at Indiana University. He received his Ph.D. in Computer Science from the University of Rochester in 2004. His research focuses on understanding human development and learning through both empirical studies and computational models.
Interactive Task Learning: A Cognitive Architecture Approach
John E. Laird
John L. Tishman Professor of Engineering
University of Michigan
Abstract
Advances in AI are leading us to a future populated with intelligent agents that have the cognitive capabilities to perform intellectually challenging tasks in health care, business, the military, and the home. However, today's agents are prisoners to the tasks for which they are programmed. Even though Deep Blue became the world's chess champion and Watson won the Jeopardy Challenge, neither of them can be taught a new task, even something as simple as Tic-Tac-Toe. In contrast, humans quickly and continuously learn new tasks throughout our lifetimes.
In this talk, I describe progress in Interactive Task Learning (ITL), where an agent learns a novel task through natural interaction with an instructor. ITL is challenging because it requires a tight integration of many of the cognitive capabilities embodied in human-level intelligence: multiple types of reasoning, problem solving, and learning; multiple forms of knowledge representations; natural language interaction; dialog management; and interaction with an external environment - all in real time. Moreover, any successful approach must be general - the agent cannot be pre-engineered with the knowledge for a given task - everything about a task has to be learned or transferred from other tasks.
Our approach builds on our research with the Soar cognitive architecture. Soar provides the fixed, task independent computational infrastructure to support the integration of cognitive capabilities underlying human-level intelligence. Our approach to ITL emphasizes mixed initiative situated interaction, where the human provides advice and information, and an embodied agent actively asks questions to acquire the knowledge it needs. I will describe how our agent (Rosie) perceives it environment, processes instructions, learns internal representations of tasks, interprets those representations to perform the tasks, and dynamically compiles its knowledge for fast task execution.
John E. Laird is the John L. Tishman Professor of Engineering at the University of Michigan, where he has been since 1986. He received his Ph.D. in Computer Science from Carnegie Mellon University in 1983 working with Allen Newell. From 1984 to 1986, he was a member of research staff at Xerox Palo Alto Research Center. He is one of the original developers of the Soar architecture and leads its continued evolution. He was a founder of Soar Technology, Inc. and he is a Fellow of AAAI, AAAS, ACM, and the Cognitive Science Society.
Organizers
Stephanie Gross, Austrian Research Institute for Artificial Intelligence, Vienna, Austria
Brigitte Krenn, Austrian Research Institute for Artificial Intelligence, Vienna, Austria
Matthias Scheutz, Department of Computer Science at Tufts University, Massachusetts, USA
Matthias Hirschmanner, Automation and Control Institute at Vienna University of Technology, Vienna, Austria